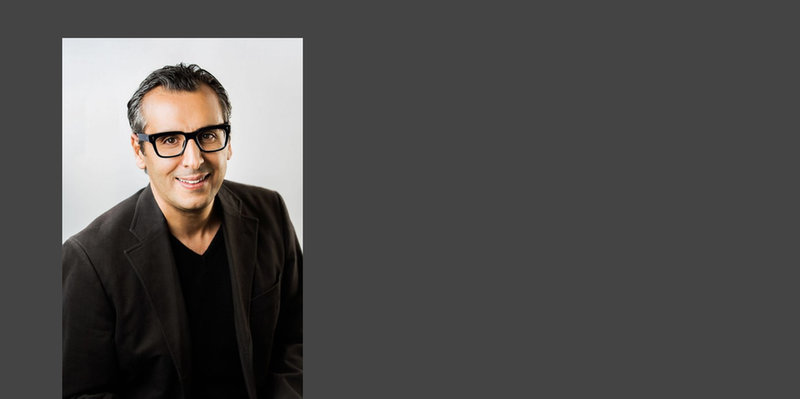
The social gradient: remote patient monitoring's new frontier
By Nassir Marrouche
Director of Cardiac Electrophysiology at the Heart & Vascular Institute at Tulane University School of Medicine
Nassir Marrouche, MD is the Director of Cardiac Electrophysiology at the Heart & Vascular Institute at Tulane University School of Medicine and founder of the Triad Research Innovation for Arrhythmia Discoveries Center (TRIAD). Prior to joining Tulane, he served as the Executive Director of the Comprehensive Arrhythmia Research and Management Center (CARMA) at the Division of Cardiology at the University of Utah´s School of Medicine. His research interests include atrial fibrillation in patients with coexisting heart failure and equitable access to wearable cardiac technology for people of lower socioeconomic status.
Telemedicine and remote patient monitoring hold vast potential for the future of cardiology, but not everyone has access to them in equal measure. And because ambulatory monitoring can have a significant positive impact on how well one manages their health, we can say that this expanding mode of delivering care has deepened the divide between the two classes of medicine we are experiencing in the U.S. and in the world. There´s telemedicine whereby a patient can log on using their smartphone or computer, look at me, and I can check for any visible signs or symptoms, and then there´s “telemedicine” that´s limited to a phone call. The majority of my patients are doing the latter because half of them cannot afford a smartphone – and that´s something we as doctors have to deal with.
It is important to keep this issue front and centre when we talk about what is probably the only positive effect of the Covid-19 pandemic, which is having accelerated the digital transformation of medicine. Without a doubt it has triggered innovation, triggered faster adoption of remotely delivered care, and proven that many in-person visits to the clinic can be done perfectly well from people´ homes. But it has also underscored the socioeconomic barriers that many of our patients face.
At Tulane University we recently started a number of partnerships with major players in medical, tech and consumer fields to explore ways to extend the reach of cardiac RPM devices to people who would benefit from them. Some of the questions we are trying to answer are, “Can we make this a part of health insurance plans?” and “Can Medicare afford to give people a $20 device that would collect a lot of relevant health information?” That is the vision of the IDEA Digital Health Center, as our new initiative is called, and that should be the vision of any health center of the future. It should target everybody, not just digitally savvy people who can afford smartwatches and fitness trackers.
As far as the technology that makes remote patient monitoring possible is concerned, it hinges on one word: wearable. Wearable this, wearable that… the word is so overused that it has nearly lost its meaning. There are wearable consumer devices that are of real value to physicians, but there are also others that are so inaccurate that they are practically worthless from a clinical standpoint.
So what is a wearable? What should we expect from a wearable? With the market for such devices becoming ever more crowded, we need a way to differentiate between them and be able to recommend to our patients which one is right for them. In the next two or three months, we are going to publish a peer-reviewed guidance paper that looks at some of these questions and proposes a set of guidelines.
Once we have clarity on what types of cardiac wearables are useful for which patients, it is up to the developer community to make sure the underlying algorithms that power the interpretation of the data work for everyone, regardless of their ethnicity, age, or the region they live in. Recently we have learned that the majority of medical AI tools in the U.S. are trained on datasets that come predominantly from three states: California, Massachusetts, and New York. And this has vast implications for how RPM tools learn and interpret data related to various health conditions and in different demographic settings
For me and my colleagues in cardiac electrophysiology, the most relevant question is: Can I read your heart story through a wearable device? To answer that question, we need to know if the device and its machine learning algorithm can reliably label data that indicate severe, moderate, and benign health states. We have a team of nurses and software engineers in place that are working to take these clinical concepts, operationalize them at the technological level, and integrate them into the patient´s situation.
Colleagues from my generation and I are lucky in that we have seen and perceived the transition phase in medicine from the analogue stethoscope model to the digital app-based remote monitoring model. Nowadays, new ideas and technologies make many things simple, but the understanding we have of how heart health and health in general reflect socioeconomic disparities can contribute greatly toward developing socially responsible medical technology. Mobile cardiac telemetry is a great place to start.
References
- Mozaffarian D, Benjamin EJ, Go AS, Arnett DK, Blaha MJ, Cushman M, Das SR, de Ferranti S, Despres JP, Fullerton HJ, Howard VJ, Huffman MD, Isasi CR, Jimenez MC, Judd SE, Kissela BM, Lichtman JH, Lisabeth LD, Liu S, Mackey RH, Magid DJ, McGuire DK, Mohler ER III, Moy CS, Muntner P, Mussolino ME, Nasir K, Neumar RW, Nichol G, Palaniappan L, Pandey DK, Reeves MJ, Rodriguez CJ, Rosamond W, Sorlie PD, Stein J, Towfighi A, Turan TN, Virani SS, Woo D, Yeh RW, Turner MB. Executive summary: heart disease and stroke statistics–2016 update: a report from the American Heart Association. Circulation. 2016;133:447–454.
- Pilkerton CS, Singh SS, Bias TK, Frisbee SJ. Changes in cardiovascular health in the United States, 2003–2011. J Am Heart Assoc. 2015;4:e001650. DOI: 10.1161/JAHA.114.001650.
- Narula S, Shameer K, Salem OMAR AM, Dudley JT, Sengupta PP. Machine learning algorithms to automate morphological and functional assessments in 2D echocardiography. J Am Coll Cardiol. 2016;68:2287–2295.
- Mayr A, Binder H, Gefeller O, Schmid M. The evolution of boosting algorithms. From machine learning to statistical modelling. Methods Inf Med. 2014;53:419–427.
- Johnson KW, Shameer K, Glicksberg BS, Readhead B, Sengupta PP, Bjorkegren JLM, Kovacic JC, Dudley JT. Enabling precision cardiology through multiscale biology and systems medicine. JACC Basic Transl Sci. 2017;2:311–327.
- Shameer K, JohnsonKW, Glicksberg BS, Dudley JT, Sengupta PP.Machine learning in cardiovascular medicine: are we there yet? Heart. 2018;104:1156–1164.
- Sengupta PP, Shrestha S. Machine learning for data-driven discovery: the rise and relevance. JACC Cardiovasc Imaging. 2019;12:690–692.
- Lancaster MC, Salem Omar AM, Narula S, Kulkarni H, Narula J, Sengupta PP. Phenotypic clustering of left ventricular diastolic function parameters: patterns and prognostic relevance. JACC Cardiovasc Imaging. 2019;12:1149–1161.
- Gandhi S, Mosleh W, Shen J, Chow CM. Automation, machine learning, and artificial intelligence in echo
- U.S. Food and Drug Administration. FDA news release: FDA permits marketing
of artificial intelligence-based device to detect certain diabetes-related eye
problems. 2018. Available at: https://www.fda.gov/news-events/pressannouncements/
fda-permits-marketing-artificial-intelligence-based- devicedetect-
certain-diabetes-related-eye. Accessed May 24, 2019.