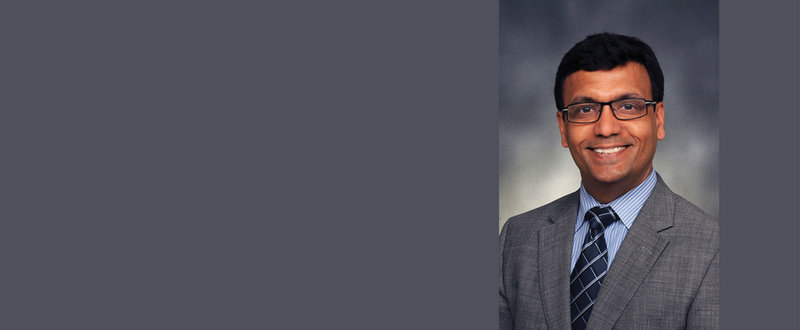
Artificial Intelligence in Cardiology
By Partho P. Sengupta,
Abnash C Jain Chair & Professor of Cardiology, WVU Heart & Vascular Institute, West Virginia University School of Medicine
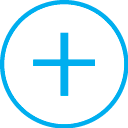
MD, DM
Partho P. Sengupta is the Director of Cardiovascular Imaging and Chair of Cardiac innovation at West Virginia University, Morgantown.
Dr Sengupta completed his clinical residency and cardiology fellowship from Mayo Clinic Rochester and Arizona respectively. He has over 160 peer-reviewed publications.
Prior to his move to WVU, he served as the Director of Interventional Echo and Cardiac Ultrasound Research at Mount Sinai Hospital, New York. He is an Associate Editor for the Journal of American College of cardiology: Imaging and has served as a Board of Director for the American Society of Echo and as the Chair of the ASE Telehealth and New Technology Taskforce.
He has won several excellence awards including ASE's Young Investigator Award in 2004, Mayo Clinic Research Award in 2007, Mayo Brother's Distinguished Fellowship Award in 2009 and AACIO Young Investigator Award in 2010. He was awarded ASE's 14th Feigenbaum Lecturership for recognizing his significant contributions to research in the field of echocardiography.
Very recently, Dr Sengupta has been named a Richard Popp Excellence in Teaching Award recipient by the American
Society of Echocardiography.
Partho P. Sengupta, MBBS, MD, DM, FACC, FASE
Abnash C. Jain Chair and chief of cardiology in WVU’s Heart and Vascular Institute and professor in the Department of Medicine, West Virginia University, Morgantown.
Artificial intelligence (AI) has begun to permeate and reform the field of medicine and cardiovascular medicine. Impacting about 100 million patients in the United States, the burden of cardiovascular disease is felt in a diverse array of demographics.1,2 Meanwhile, routine mediums such as multimodality images, electronic health records (EHR), and mobile health devices store troves of underutilized data for each patient. AI has the potential to improve and influence the status quo, with capacity to learn from these massive data and apply knowledge from them to distinct circumstances.3,4 With considerable information in each heart beat, cardiovascular medicine will definitely be one of the fields that embrace AI to move toward personalized and precise care.5
AI has already been woven into the fabric of everyday life. From an internet search engine, email spam and malware filtering, to uncovering fraudulent credit card purchases, AI addresses an individual’s needs in the realms of business, entertainment, and technology. Unfortunately, medicine, including cardiology, has not fully embraced this revolution, with only a limited number of AI-based clinical applications being available. Nevertheless, there is promise towards routine implementation; machine learning and deep learning have seen an exponential surge of cardiovascular publications in the past decade.6,7 These methods have proven beneficial in a variety of complex areas including echocardiogram interpretation and diastolic dysfunction grade stratification.8,9
The US Food and Drug Administration has already approved several devices that utilize AI features.10 Imagine coming to work finding that your system has analyzed all your patients while you were sleeping: their laboratory data, imaging results, symptoms, and mobile device data to calculate their risk of cardiovascular events, death, hospitalization, whether medications should be adjusted/added/removed, or whether they should be referred for an examination. The system presents you with the reasons for its recommendations, and you are confident that they are as good as those given by the most experienced physicians. This may allow you to spend time in shared decision making with your patients, both objectively and compassionately. Although there are currently several barriers/challenges to adoption of AI in clinical practice, undoubtedly, AI will drive current healthcare practice towards a more individualized and precision-based approach over the next several years. Therefore, general understanding of AI techniques by clinicians and researchers in cardiovascular medicine is paramount.
AI has emerged as a promising tool in cardiovascular medicine. With the popularization of big data and machine power, the fundamentals of healthcare practice and research are bound to change. Traditional statistics remain highly effective in a simple data set and in assessing causal relationship; however, many areas in clinical practice and research will be led by powerful prediction and exploration of big data using AI. Particularly, the capability of AI to analyse unstructured data expands the field of cardiovascular research. In addition, AI will further increase its contribution to mobile health, computational modeling, and synthetic data generation, with new regularizations for its legal and ethical issues. In this paradigm shift, deep understanding of physiology and disease mechanisms remains paramount to interpret the results of AI. Meanwhile, AI literacy will become essential to understand the latest medical knowledge and to use novel medical devices.
References
References
- Mozaffarian D, Benjamin EJ, Go AS, Arnett DK, Blaha MJ, Cushman M, Das SR, de Ferranti S, Despres JP, Fullerton HJ, Howard VJ, Huffman MD, Isasi CR, Jimenez MC, Judd SE, Kissela BM, Lichtman JH, Lisabeth LD, Liu S, Mackey RH, Magid DJ, McGuire DK, Mohler ER III, Moy CS, Muntner P, Mussolino ME, Nasir K, Neumar RW, Nichol G, Palaniappan L, Pandey DK, Reeves MJ, Rodriguez CJ, Rosamond W, Sorlie PD, Stein J, Towfighi A, Turan TN, Virani SS, Woo D, Yeh RW, Turner MB. Executive summary: heart disease and stroke statistics–2016 update: a report from the American Heart Association. Circulation. 2016;133:447–454.
- Pilkerton CS, Singh SS, Bias TK, Frisbee SJ. Changes in cardiovascular health in the United States, 2003–2011. J Am Heart Assoc. 2015;4:e001650. DOI: 10.1161/JAHA.114.001650.
- Narula S, Shameer K, Salem OMAR AM, Dudley JT, Sengupta PP. Machine learning algorithms to automate morphological and functional assessments in 2D echocardiography. J Am Coll Cardiol. 2016;68:2287–2295.
- Mayr A, Binder H, Gefeller O, Schmid M. The evolution of boosting algorithms. From machine learning to statistical modelling. Methods Inf Med. 2014;53:419–427.
- Johnson KW, Shameer K, Glicksberg BS, Readhead B, Sengupta PP, Bjorkegren JLM, Kovacic JC, Dudley JT. Enabling precision cardiology through multiscale biology and systems medicine. JACC Basic Transl Sci. 2017;2:311–327.
- Shameer K, JohnsonKW, Glicksberg BS, Dudley JT, Sengupta PP.Machine learning in cardiovascular medicine: are we there yet? Heart. 2018;104:1156–1164.
- Sengupta PP, Shrestha S. Machine learning for data-driven discovery: the rise and relevance. JACC Cardiovasc Imaging. 2019;12:690–692.
- Lancaster MC, Salem Omar AM, Narula S, Kulkarni H, Narula J, Sengupta PP. Phenotypic clustering of left ventricular diastolic function parameters: patterns and prognostic relevance. JACC Cardiovasc Imaging. 2019;12:1149–1161.
- Gandhi S, Mosleh W, Shen J, Chow CM. Automation, machine learning, and artificial intelligence in echo
- U.S. Food and Drug Administration. FDA news release: FDA permits marketing
of artificial intelligence-based device to detect certain diabetes-related eye
problems. 2018. Available at: https://www.fda.gov/news-events/pressannouncements/
fda-permits-marketing-artificial-intelligence-based- devicedetect-
certain-diabetes-related-eye. Accessed May 24, 2019.
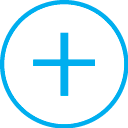