Why does cardiology need artificial intelligence?
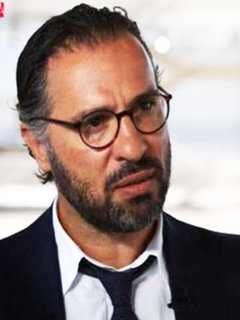
Thomas Modine, MD PhD
Authors: W. Ben-Ali MD Ph.D1,2, P. Ouvertchouk MD1, A. Sudre MD1, T. Modine MD PhD
- Structural valve program, CHRU-Lille, Lille, France
- Department of cardiac surgery, Montreal Heart Institute, Montréal, Canada
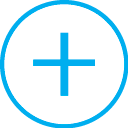
Thomas Modine graduated from Lille University Hospital, France. He has MD, PhD and MBA degrees and is a renowned surgeon in TAVI, TMVR, minimally invasive surgery and valve sparing techniques. He is also involved in basic science research programmes and is developing a percutaneous mitral programme with IP. From 2004 to 2006, Thomas completed a PhD thesis and then went on to complete a 1-and-a-half-year fellowship at Harefield Hospital, London. Later he dedicated his training to the development and progress of percutaneous techniques and heart team approach. His research interests are in aortic valve complex, bioengineering, beating heart surgery, hybrid approaches, TAVI and TMVR. Both basic and clinical aspects of research experiences are studied. He is currently working as a consultant cardiac surgeon at Lille University Hospital and professor at Jiao Tong University, Shanghai.
Thomas Modine, MD, PhD, MBA
Cardiothoracic Surgeon at Centre Hospitalier Régional Universitaire de Lille, Lille, France
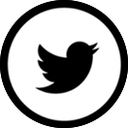
The concepts of artificial intelligence (AI) was firstly reported in a publication of Alan Turing in 19371. The term AI was used for the first time at the Dartmouth Conference in 19562. AI is the product of the combination of sophisticated mathematical models and computation, which allows the development of complex algorithms capable of emulating human intelligence. The recent advances in computing power and the improvement of the quality of data allowed the expansion of Machine Learning (ML) algorithms.
In cardiology, ML algorithms allows automated analysis and interpretation of data from, among others, electronic health records, electrocardiography, echocardiography computed tomography, magnetic resonance imaging, and yield to high-performance predictive models supporting decision-making that can improve diagnostic and prognostic performance.
Several application of ML predictive models in cardiology were reported, predicting mainly: the deterioration of ventricular function in patients with repaired tetralogy of Fallot using support vector machine3, the risk of occurrence of cardiovascular events using naïve Bayes fusion and genetic algorithm4, survival using clinical and electrocardiographic variables with a random forest method5, occurrence of cardiac arrest using artificial neural networks6, bleeding after percutaneous coronary intervention using gradient boosting method7 and more recently survival after transcatheter aortic valve replacement using data provided by the open-access National Inpatient Sample database8.
ML-based predictive systems have major limitations: interpretability and overfitting. Building predictive models is inherently based on past events, and the future will not necessary resemble the past, nor will these models necessary perform well in a population different from the one represented in the training cohort9.
There were also challenges for the clinical implementation of these models: ethical limits of use, data security, quality of data or healthy data, dealing with errors and staff remuneration concerns fearing the ability of these algorithm to replace physicians. Barone Rochette10 stated that AI will probably change the work of cardiac imaging specialists. However, physicians will remain the final decision-maker in medical management on the basis of all elements of the patient’s medical report.
In conclusion, several successful applications of AI in cardiology has been reported allowing significant improvements in diagnosis, therapeutic and prognosis point of views. Healthy data are mandatory for the success of these algorithms. However, AI have to face several challenges for its implementation, specially, ethical limits and fear of the change of physician implication in the patient care. Therefore, physician should strongly be involved in the development of this tool in order to integrate it adequately into diagnosis and therapeutic strategies.
References
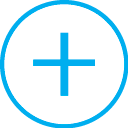
References
- Turing AM. On comutable numbers, with an application to the Entscheidungs problem. Proc Lond Math Soc 1937;42:230–65.
- MooreJ. TheDartmouth College Artificial Intelligence Conference: The Next Fifty Years. AI Magazine. 2006;27(4):87-91.
- Samad MD, Wehner GJ, Arbabshirani MR, Jing L,Powell AJ ,Geva T,et al. Predicting deterioration of ventricular function in patients with repaired tetralogy of Fallot using machine learning. Eur Heart J Cardiovasc Imaging. 2018;19(7):730-8.
- Paredes S, Rocha T, de Carvalho P, Henriques J, Morais J, Ferreira J. Integration of different risk assessment tools to improve stratification of patients with coronary artery disease. Med Biol Eng Comput. 2015;53(10):1069-83.
- Samad MD, Ulloa A, Wehner GJ, Jing L, Hartzel D, Good CW, et al. Predicting survival from large echocardiography and electronic health record datasets: optimization with machine learning. JACC Cardiovasc Imaging. 2019;12(4):681-9.
- Kwon JM, Lee Y, Lee S, Park J. An algorithm based on deep learning for predicting in-hospital cardiac arrest. Am Heart Assoc. 2018;7(13): pii: e008678.
- Mortazavi BJ, Bucholz EM, Desai NR, Huang C, Curtis JP, Masoudi FA, et al. Comparison of machine learning methods with national cardiovascular data registry models for prediction of risk of bleeding after percutaneous coronary intervention. JAMA Netw Open. 2019;2(7):e196835.
- Hernandez-Suarez DF, Kim Y, Villablanca P, et al. Machine learning prediction models for in-hospital mortality after transcatheter aortic valve replacement. J Am Coll Cardiol Intv 2019;12: 1328–3
- Chen JH, Asch SM. Machine learning and prediction in medicine—beyond the peak of inflated expectations. N Engl J Med 2017;376: 2507–9
- Barone-Rochette G. Will artificial intelligence change the job of the cardiac imaging specialist? Arch Cardiovasc Dis (2019), https://doi.org/1016/j.acvd.2019.11.002