Artificial Intelligence in Nuclear Cardiology
By Piotr Slomka,
PhD, FACC
Director of Innovation in Imaging, AI in Medicine Program, Department of Medicine, Cedars-Sinai
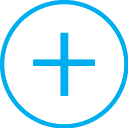
Piotr Slomka, PhD, FACC, is the Director of Innovation in Imaging in the Artificial Intelligence in Medicine Program within the Department of Medicine and Professor of Medicine at Cedars-Sinai, as well as Professor of Medicine In-Residence at the David Geffen School of Medicine at UCLA. He received his doctorate in Medical Biophysics in 1995 from the University of Western Ontario, Canada. Slomka was elected as a fellow of the American College of Cardiology in 2008.
Slomka's research aims to improve the diagnosis and risk assessment of heart disease by developing novel software methods and image-processing algorithms. His research focuses on development of practical clinical innovations and new approaches to quantification of perfusion, ischemia, image registration, multimodality imaging and machine learning, impacting the clinical practice of cardiovascular SPECT and PET. Currently, Slomka is the principal investigator of two National Institutes of Health R01 grants:
- Automated fast single-photon emission computed tomography-myocardial perfusion imaging
- 18F-fluoride positron emission tomography and computed tomography angiography
As of 2020, Slomka has had more than 315 publications in peer-reviewed journals. He serves as the faculty member for national societies, including Society of Nuclear Medicine and American Society of Nuclear Cardiology and as an associate editor for the Journal of Nuclear Cardiology.
Piotr Slomka, PhD, FACC
Director of Innovation in Imaging, AI in Medicine Program, Department of Medicine, Cedars-Sinai
Single photon emission computed tomography (SPECT) or positron emission tomography (PET) myocardial perfusion imaging (MPI) for the diagnosis and management of coronary artery disease (CAD) are common imaging modalities for assessment of coronary artery disease. I believe artificial intelligence (AI) represents an optimal approach to integrating and analyzing the vast amount of clinical, stress, and imaging data available with each nuclear cardiology scan. Currently, even experienced clinicians can struggle with accurately interpreting and integrating all the information. While nuclear cardiology analysis has been robustly automated using traditional image processing approaches, AI can further improve cardiovascular image analysis by directly extracting and integrating large volumes of information to improve diagnostic and prognostic accuracy.
Large, well-defined databases are key for the development and successful implementation of all AI-based applications. We have established a large multi-center registry—REgistry of Fast Myocardial Perfusion Imaging with NExt generation SPECT (REFINE SPECT); to date, over 24,000 complete SPECT MPI datasets from 9 international centers have been collected with over 290 perfusion and function features automatically extracted from each study and merged with clinical variables. In the diagnostic cohort, the results of invasive angiography and revascularization were also obtained; in the prognostic cohort, follow-up was obtained for major adverse cardiovascular events (MACE). REFINE SPECT provides diverse and comprehensive data that is demonstrative of a more globally representative population and ideal for training AI-based algorithms for image processing, disease diagnosis, and risk prediction.
To ensure the clinical utility of SPECT or PET MPI, accurate image analysis (e.g. localization, segmentation, quantification) are critical. AI-based algorithms can improve the precision of this process while also reducing the need for time-consuming manual adjustments. Our team at Cedars-Sinai Medical Center proposed a fully automated machine learning algorithms for more accurate image segmentation, disease detection and prediction of risk for adverse outcomes.
While classical machine learning assesses existing observations based on expert engineered features to more accurately predict future outcomes, new deep learning AI methods can learn directly from the image data further improving image analysis. In the first nuclear cardiology application of deep learning, our group demonstrated a direct interpretation of SPECT MPI for the diagnosis of obstructive CAD. Additionally, in a separate study we demonstrated that DL can be applied to automatically combine upright and supine MPI polar maps and to predict obstructive CAD. In clinical practice, physicians need to review these two images for the final interpretation to mitigate various imaging artifacts, such as attenuation artifacts. The deep learning method was applied to SPECT polar map images and improved the automatic prediction and localization of obstructive CAD compared with current methods – importantly these results can be obtained retrospectively without any change in equipment, protocol, or radiation exposure for patients.
A key factor in deciding about the clinical acceptance of these new AI method is the ability to explain the AI findings to the physicians. The traditional perception of AI as a “black box” can be overcome by presenting the rationale for the computed decision or recommendation. This can be done through highlighting image or clinical features and presenting the AI-based prediction through attention maps and individualized patient-specific explanations of risk. AI should not be viewed as a replacement for the physician but rather to serve as an aid in interpreting the nuclear cardiology scans and improving the accuracy of the reads. As the field of nuclear cardiology continues to evolve, I expect AI to play a key role in this evolution, through the integration of AI-based analysis with routine clinical interpretation of nuclear cardiology scans.
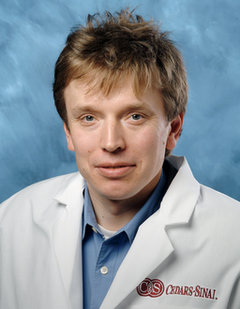
As the field of nuclear cardiology continues to evolve, I expect AI to play a key role in this evolution, through the integration of AI-based analysis with routine clinical interpretation of nuclear cardiology scans.
Piotr Slomka, PhD, FACC